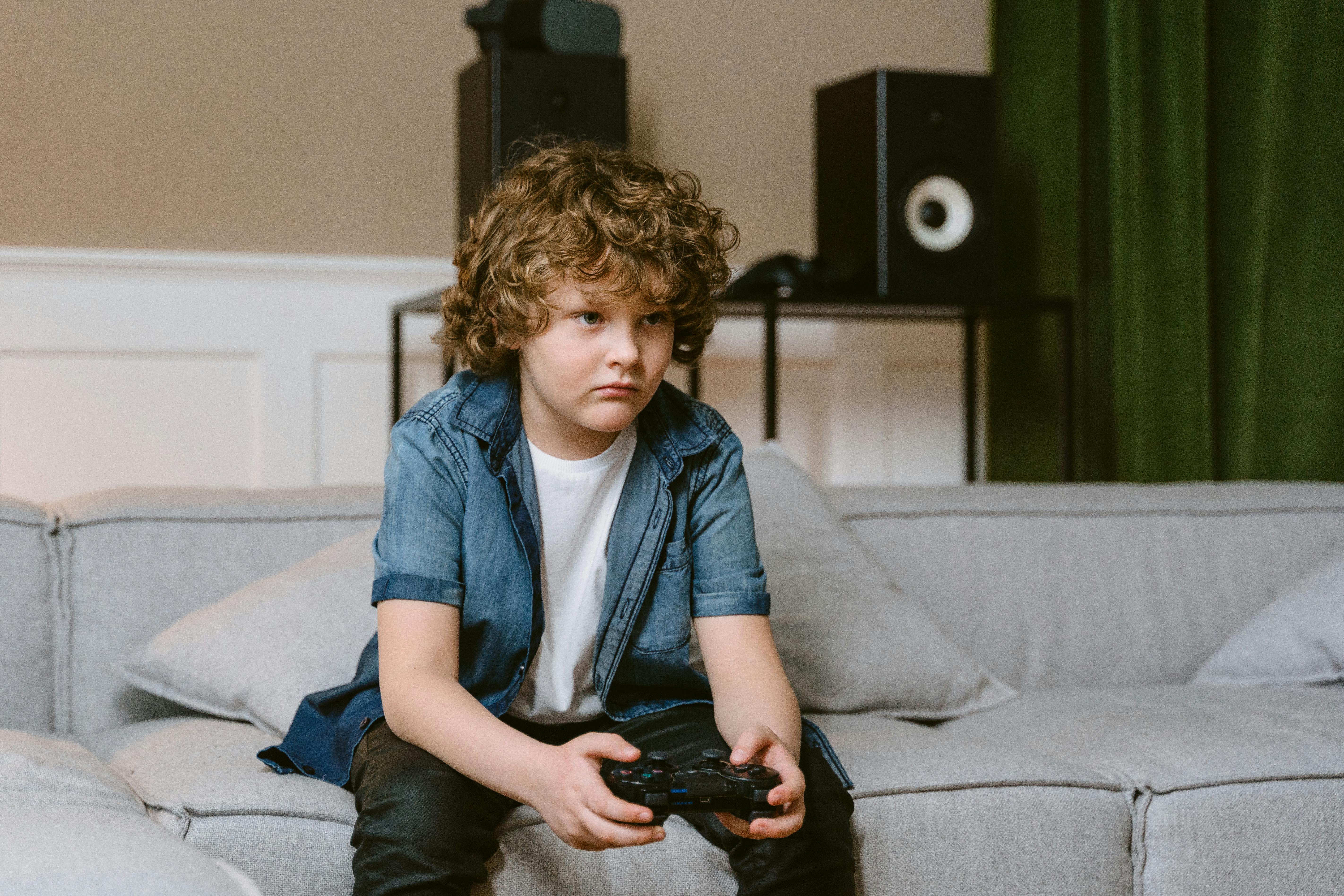
Data science process
Data science as a field sounds simple, there is data and science is used to find meaning in data. But it is not that simple in practice. The volume of data collected is not in its simplest form, it is generally unstructured and raw, and the tools used require expert knowledge. It can be said that the entire flow of data science in a data product is a complex, technical process that needs training and practice.
WHY IS DATA ANALYSIS NEEDED?
Data science is a domain that requires skills in mathematics, statistics, and computer software and programming. Data science uses sophisticated models to find valuable information. It is a field that has entered all other industries with great speed. Every day, various data scientists find solutions to problems posed by the market, the business environment, customers, and customers. So why do these companies have such an urgent need for analytics and how do you help them?
-
Helps to understand the customer and their needs from sale to post-purchase satisfaction.
-
Helps in marketing and understanding marketing trends and opportunities.
-
Helps to optimize production, operation, human resources, etc. to improve the performance of the business unit.
-
It helps in branding and communicating with the outside world, and makes one’s business visible through digital marketing and social media marketing.
-
It helps in innovation and experimentation in real time, which in turn saves a lot of time and effort.
Therefore, it can be said that data science increases business value and helps to compete with other players effectively.
WHAT DO DATA SCIENTISTS DO?
This is one of the main questions asked: what does a data scientist do in his day?
- Frame a problem: To frame a problem, you need to understand the goals of the person whose project you are managing. What do you want to achieve and what are the obstacles? The problem must be clear and simple, and not aggravated, since it is the stepping stone and without a problem, one will have no direction.
- Raw data collection: depending on the problem, it is necessary to obtain all the data that includes the variables in question. Data can be collected from internal databases or purchased from external data sets.
- Process the data for analysis– The data collected is often raw and unstructured, especially if it is not well maintained. To analyze your data, you need to make sure that all errors and errors such as missing values, data range errors, time zone differences, and invalid inputs are cleaned up and corrected.
- Explore the data: This is also called exploratory data analysis (EDA), more like playing with the data. Analysts must prioritize the questions they want to ask and search the data. The data has many trends and patterns hidden in it, the job of the analyst is to identify those patterns that can be turned into information.
- Machine learning and algorithm construction: This is the deep visualization and exploration step; here the scanned data is used to create a story. The data is subjected to various mathematical and statistical tools and programs to find meaning. The data is used as input for different predictive analytics algorithms.
- Communicate results: The knowledge that is gathered must be interpreted and communicated to management professionals, it is like telling stories in such a way that people without technical knowledge can understand. Proper presentation of results will lead to decision making and timely action.
Data scientists have a challenging role, as they are now people who find problems and also the means for their solutions.